The success of your ecommerce platform hinges entirely on offering a convenient and expedient user experience. However, messy data can get in the way. Seemingly trivial inconsistencies like not having naming conventions or tags can make it much harder for customers to find products or access the right information. When this happens, they will quickly move on.
Before we discuss some solutions, let’s define what we mean by “messy” data.
- Kept in silos — Data from one source cannot be easily connected with data from another source. Social media advertising analytics live on one platform, while sales data lives on another, so you have no way of determining the return on ad spend, much less understanding which social media platforms bring the highest return on investment. This can result in misallocated budgets and wasteful spending.
- Inconsistent — A lack of naming conventions results in messy data that is difficult to index through a semantic query. For example, if one person labels a “notebook” as having “wifi” capabilities while another refers to a “laptop” with “wireless LAN” the database treats this as a duplicate item. If a customer uses a “notebook” web filter to search for an item labeled “laptop” that item won’t show up in their query and the sale won’t be made.
- Unstructured — Product data isn’t broken down into indexable attributes. For example, the text description simply reads “cordless lawn mower rechargeable battery 40 volts.” This text description contains four attributes describing the item. However, in its present form, it cannot be used for any kind of application requiring structured data, such as website filtering, a digital assistant, or product recommenders.
- Incomplete — Having an incomplete dataset makes it difficult (if not impossible) to extract insights from your data. For example, if you don’t have sentiment analysis data from every customer contact channel, how can you determine which customers are at the highest risk of churn?
Semantics as a solution to messy data
Semantics are a form of metadata (data about your data) that describes the meaning of your data, such that everyone in your organization knows how to properly label, index, and find the information they need. Simply put, it’s a guiding principle for formatting your data in universal terms and determining what goes where based on a clear hierarchy. However, semantics can also help you establish links between, say, your CRM and sales data, and derive even more meaning from your customer profiles.
Not only can you see a customer’s purchase history, but you can calculate their lifetime value and see which product recommendations resulted in an upsell, or create new customer segments based on similar content consumption patterns.
Semantics is about connections
Every piece of data can be semantically enriched to increase its context. In the example above, you can derive the who, what, when, where, why, and how of your data simply by linking two formerly siloed databases to tell a clearer story of who your customers are and what they want.
While semantics is undoubtedly an abstract concept, in practice, it’s similar to the formation of neural connections in the brain. When humans learn something new, the brain forms new pathways between neurons (nerve cells), thereby enhancing information retention.
In the context of ecommerce, that means enhancing the meaning of each piece of data, which leads to better product recommendations, greater opportunities for upselling, and easy-to-use self-service solutions for customers. For example, linking your knowledge base with your chatbot means that when a customer requests information on how to execute a particular task in your software product, the chatbot points them to a corresponding how-to guide from your knowledge base.
How do you create better connections in your data?
Standardization and linking information are key when it comes to cleaning up your data. This entails establishing a company-wide framework for how you format your metadata. Start with the following items:
- Ontology — Ontology refers to how information is organized. An example of this is a sitemap, which shows how web pages are indexed within a menu and linked to one another in a logical order. When it comes to semantic search, this organizing framework looks more like a tree with nodes branching from it. Each node represents an organizing principle, such as a product category.Beneath that product category are attributes such as color, features, related accessories, and so on. For example, under ‘houseware’ you have ‘kitchen appliances’ and beneath that ‘blenders’ and so on.
Ontology breaks down and connects data attributes to make sense of products. An effective semantic search powered by ontology is designed based on customer intent rather than internal hierarchies. If a customer inquired about “mixers” rather than ‘blenders’ the ontology would recognize the searcher’s intent and show them the corresponding products. The nodes in the ontology have synonyms for each term such that the same search results are triggered for different natural language representations of the same search term.
- Tags — Tagging product descriptions, knowledge articles, video content, and more makes them easier to find when a user inputs a search term. However, more tags aren’t necessarily better. Instead, agree on a set of five or six tags for a specific product line or series of landing pages and use those tags exclusively, which takes the guesswork out of search.
- Naming conventions — Standardize the way you categorize and name products. For example, if one person describes an item as “blue” while another inputs “aquamarine”, the database may treat this as a duplicate item. Also, agree on how you’ll denote units of measurement. Is “520 MB” the same as “0.5 gigabytes”?
- Word definitions — If you use the same word in a different context, the definition changes. For example, the word ‘feedback’ means something different when referencing ‘audio feedback’ versus a ‘feedback loop.’ Make sure to distinguish the meanings of terms that occur in more than one context.
- Product descriptions — Make sure your product descriptions reflect the naming conventions you’ve used in your database. For example, if the product name says ‘smart speaker’ while the product description mentions ‘voice assistant’, customers may be confused.
- Linked data — Linked data is structured data that is interlinked with other data so that it becomes more useful in semantic queries. For example, showing a customer complementary product recommendations (eg: a pair of shoes to go with the dress they just bought) is only possible with linked data, and is a key tool for cross-selling and upselling. Linking a product with corresponding accessories, services or upgrades helps increase purchase size.
- Internal file sharing — Companies often have terms that are exclusively used for internal purposes. When the naming conventions in internal documents don’t match your customer-facing collateral, this can result in confusion or misinterpretation.
What are the benefits of using a semantic data model?
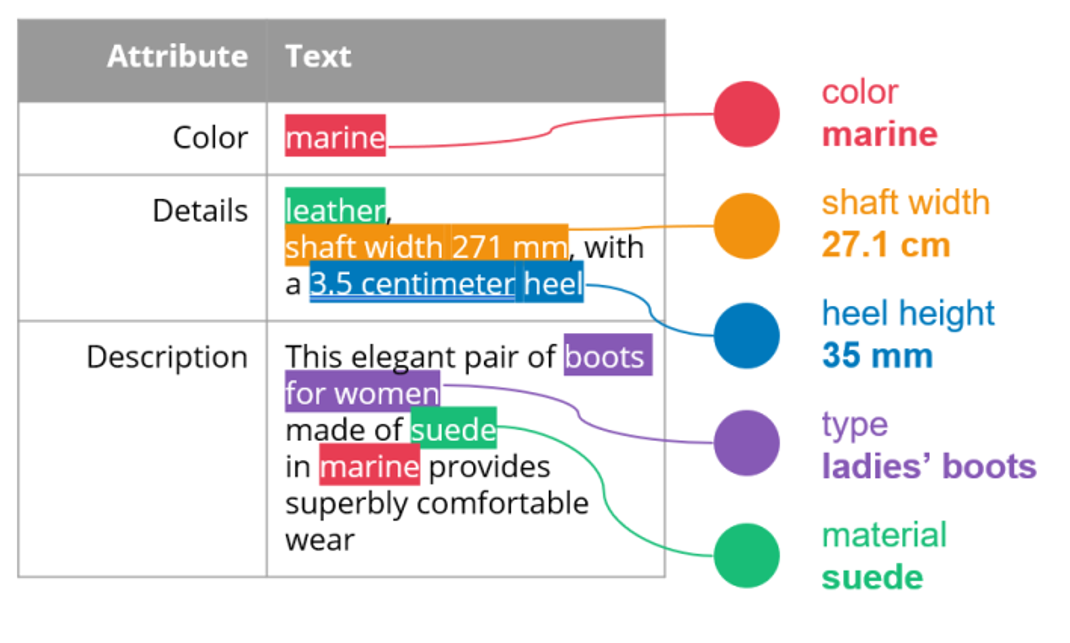
1. Enables businesses to drive meaning
Without semantics, data is pure syntax. For example, consider this dataset: “Brand new; good condition; mint condition; needs some repair.” Each of these terms is relative. If you sell second-hand items, clearly defining these terms is important so that customers know what they’re buying. Displaying a corresponding chart that shows what each term means helps customers make better decisions while making it easier on the back-end to assign the right categories to each product.
2. Improves findability within site search
Labeling your data according to the searcher’s intent means that even if customers don’t know what they’re looking for, they will still be able to find it. Say you sell a niche product, such as a lens extender for DSLRs. Those who are new to photography will probably search for “lens accessories” or “lens enhancers.” Making your products searchable using synonyms based on natural language vastly improves product discoverability.
3. Helps drive relevancy
Linking products under a common hierarchy makes it possible to display relevant product recommendations like ‘You may also like…’ Finally, properly indexing your content — especially your knowledge base — empowers customers to find their own solutions rather than having to contact customer support for every little problem.